Upcoming Events:
Tuesday, 4 February, 10:30 am
Book Talk, “The Paradox of Smalltown Crime”, Attic Angel, 8302 Old Sauk Rd., Middleton, Wis.
Thursday, 20 March, 5-7 pm
Tomah Chamber of Commerce Author Showcase, Three Bears Resort, 701 Yogi Circle, Warrens, Wis.
Subscribe to my YouTube Channel
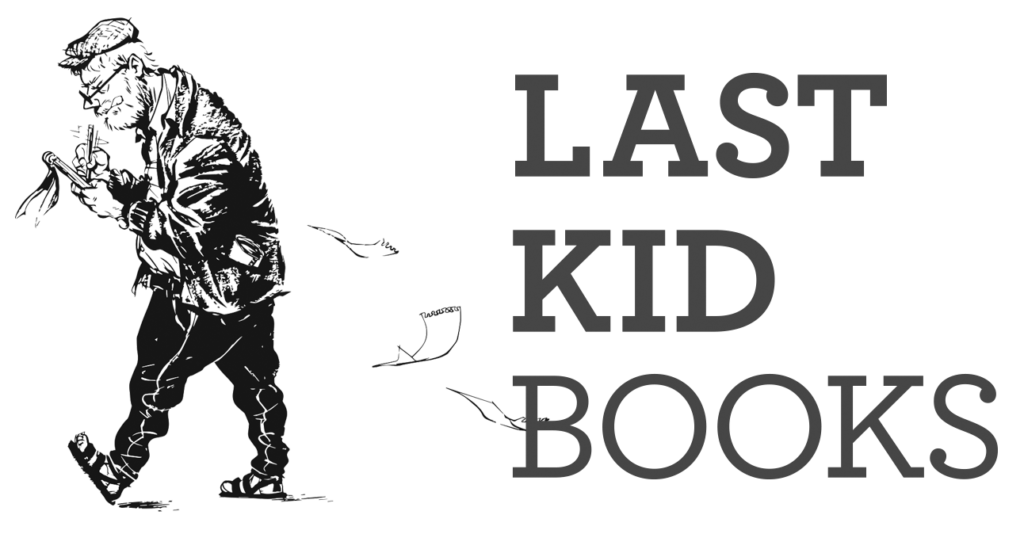
The Law of Diminishing Informative Returns
by David Benjamin
“These [AI] methods only work in areas where things are empirically true, like math and science. The humanities and the arts, moral and philosophical problems are much more difficult.”
— Dylan Patel, chief analyst, SemiAnalysis,
MADISON, Wis.—For most of my career, I’ve been a slave to technology. This bondage began in earnest with the transition in the printing industry from the Rube Goldberg magnificence of the Linotype machine to “cold type.” I suffered this trauma as manager of a publications company where the copy for all my periodicals emerged from a “Compugraphic” machine in the form of a white strip of ticker tape punched with polka dots in infinite combination. My typist could actually read those little holes and translate them into English.
I never mastered that skill, nor did I try. I’ve transitioned, grudgingly, into the digital era but have obstinately tightened my grip on the analog sensibilities that have sustained me while also—not coincidentally—enriching my insight into the symbiosis of technology with the “human element.”
Perhaps the most powerful—and unintentionally ironic—human element in “high tech” is a sort of death wish among the industrialists of the “digital revolution.” They yearn Muskishly to eliminate mortal involvement in virtually all facets of the economy. They dream of turning over every saleable task, mental and muscular, to machines possessed of an omniscient “artificial intelligence” (AI).
I experienced a conundrum of this drama on route from a holiday gathering. Beside me in the car was Hotlips, whose metier has been, for decades, high-tech journalism. I owe to her my tenuous grasp of digital stuff, including the recent push among tech and auto companies to pump into cars so much AI that steering wheels will vanish and tomorrow’s horseless carriages will drive themselves.
This mission has faltered somewhat. Trials of “autonomous vehicles” (AV) have ranged from barely satisfactory to—especially in the case of Tesla’s “Full Self-Driving” (FSD) feature—homicidal. But it’s not in fiascos like the Ford robotaxi that failed to “sense” the presence of a fallen pedestrian on the street until it had dragged her along the pavement for twenty-odd abrasive feet, but in subtleties that clarify the gap between the human element and “machine learning.”
Christmas traffic on I-90 was light, but distinctly last-minute. Drivers were in a hurry to reach home, hearth and egg nog. As I approached a car moving more slowly than mine and prepared to pass, I checked my rearview. There, just before it entered my left-shoulder blind spot, was an SUV topping ninety miles an hour.
The SUV flew by. I didn’t bother to look back again. I just said to myself, “Wait for it.” And there he came. Ten seconds later, out of “nowhere,” a trailing vehicle, this time a souped-up minivan with Illinois plates, roared past in hot pursuit of the breakneck SUV. I long ago figured out that psychotic speeders travel in pairs, locked in a de facto drag race whose mirage—at the end of the highway or in a ditch beside it—is their manhood. My awareness of this syndrome is the sort of intuition that cannot be programmed, in the current state of the art, into a “self-driving” car. No AV could anticipate the peril posed by a pair of morons in a bumper-to-bumper chickie run in holiday traffic on an interstate highway.
My hesitation to change lanes, before the sudden arrival of Maniac #2, was a peculiarly analog response derived from a lifetime of defensive driving. Even if I had pulled out, my reflexes might have yet prevented a crash. An AV, in the same dilemma, might have also reacted effectively. But only a person—me—had the street smarts to pause, lament the sheer stupidity of my fellow man, and let the threat pass without need of an emergency response.
As we crossed from Monroe to Juneau County, I mentioned to Hotlips the change in the highway surface. Between our Tomah departure and our destination in Madison, we encountered stretches of concrete both smooth and fluted, surfaces that appeared to be predominantly asphalt and sections where repaired patches of asphalt indicated a random range of paving materials.
With each change, I had to heighten or relax my vigilance because, twenty-four hours before, I-90 had been him by a “wintry mix” of sleet, freezing rain and snow. The road looked smooth and dry but I didn’t trust it, especially on stretches streaked with a white substance that might be salt or frost, and marked by dark patches that might be “black ice,” the deadliest hazard of winter driving. “Eyeballing the road,” like reading other drivers, is a nuance not easily translated into a series of ones and zeros. Indeed, the vast majority of my fellow drivers, ripping down the road at ninety per, were clearly oblivious to the subtle differences—and their effect on safety—between, for example, tarmac, hot mix asphalt and concrete, either smooth or scored. You can save your life by reading the road, but you can’t teach this skill to most people, much less a machine.
Why can’t AI do this?
The data required to infuse an electronic control unit (ECU) with this level of “feel” is beyond the experience of most human programmers and so sophisticated that the machine-learning program would be immense. At this time—and, I think, in the foreseeable future—it is inconceivable within any known database.
I’m interested in the difficulty of translating street smarts into artificial intelligence because this has become a focus of Hotlips’ reporting. Despite rosy predictions by the gods of Silicon Valley, she has argued that AI is not the digital panacea that will end all drudgery and usher humanity into a cushy retirement waited upon by robots that eerily resemble Daryl Hannah. Lately, indeed, I’m reading about what might be called the Law of Diminishing Informative Returns. AI is running out of data to feed its appetite for more and more stuff to “know.”
Simply expressed, AI “learns” by gathering massive amounts of information—more than humanly possible but indiscriminate—from every possible digital source. This empowers AI to answer any question asked … or to make one up. The euphemism for a lie told by AI is “hallucination.”
For example, Hotlips recently used ChatGPT to generate my bio. In less than 290 words, AI told eighteen lies about me, including the “facts” that I’m a Long Island Jew with bylines in the New York Times and Esquire. AI also gave me a Pulitzer Prize and credit for writing and producing “The Sopranos” on HBO.
Among the dirty secrets secrets of AI is the immense power consumption needed to compile whoppers like my fake-news bio. The energy and resources devoured by data centers processing “generative AI”—and cryptocurrency, which is a whole other nightmare—is so profligate that surrounding neighborhoods are subject to electrical blackouts and datacentric droughts. People in Arizona are buying bottled water from France because AI is sucking the Colorado River dry.
And, of course, these data centers are fomenting, from their colossal ingestion of unfiltered “content,” lies and errors that go uncorrected—and often unnoticed—by human intervention, and without the sort of neurotic eyeball scrutiny that I apply to I-90 in the wintertime.
AI, today, is a hoarder. As anyone knows who has ever hunted coins, stamps, butterflies or bottle caps, collecting is easy. It’s organizing the collection that gets harder as it gets bigger, and the Law of Diminishing Informative Returns applies.
AI without vigilance is like the old guy down the block with a houseful of books, tumbling from shelves, piled on windowsills, stacked on the floor and coated with dust. The collector needs to look up something but where—and which book is right? On the other end of the block: a public library. It might indeed contain fewer books, but it has the Dewey Decimal System—and librarians.